Statistical Inference and Modeling for High-throughput Experiments - November 2024
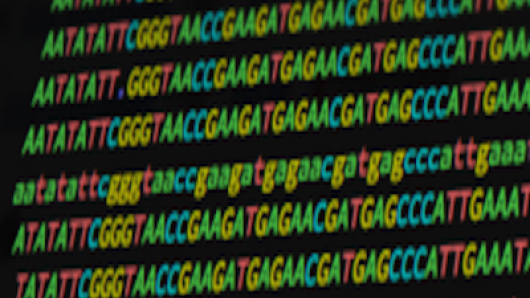
- Intermediate
Associated Schools
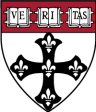
Organizing high throughput data
Multiple comparison problem
Family Wide Error Rates
False Discovery Rate
Error Rate Control procedures
Bonferroni Correction
In this course, you’ll learn various statistics topics including multiple testing problems, error rates, error rate controlling procedures, false discovery rates, q-values, and exploratory data analysis. We then introduce statistical modeling and how it is applied to high-throughput data. In particular, we will discuss parametric distributions, including binomial, exponential, and gamma, and describe maximum likelihood estimation. We provide several examples of how these concepts are applied in next-generation sequencing and microarray data. Finally, we will discuss hierarchical models and empirical Bayes along with some examples of how these are used in practice. We provide R programming examples in a way that will help make the connection between concepts and implementation.
This class was supported in part by NIH grant R25GM114818.